#使用 PyTorch 实现线性回归模型的代码
import torch
import torch.nn as nn
import matplotlib.pyplot as plt
# 生成模拟数据
torch.manual_seed(42)
x = torch.linspace(-1, 1, 100).view(-1, 1)
y = 2 * x + 1 + 0.3 * torch.randn(x.size())
# 定义线性回归模型
class LinearRegressionModel(nn.Module):
def __init__(self):
super(LinearRegressionModel, self).__init__()
self.linear = nn.Linear(1, 1)
def forward(self, x):
return self.linear(x)
model = LinearRegressionModel()
# 定义损失函数和优化器
criterion = nn.MSELoss()
optimizer = torch.optim.SGD(model.parameters(), lr=0.01)
# 训练模型
epochs = 1000
for epoch in range(epochs):
optimizer.zero_grad()
outputs = model(x)
loss = criterion(outputs, y)
loss.backward()
optimizer.step()
if (epoch + 1) % 100 == 0:
print(f'Epoch [{epoch + 1}/{epochs}], Loss: {loss.item()}')
# 进行预测
with torch.no_grad():
predicted = model(x)
# 绘制结果
plt.scatter(x.numpy(), y.numpy(), label='True data')
plt.plot(x.numpy(), predicted.numpy(), 'r', label='Predicted')
plt.legend()
plt.show()
'''
在这段代码中,首先生成了模拟的线性数据,并添加了一些噪声。
然后定义了一个线性回归模型,包含一个线性层。
接着使用均方误差损失函数和随机梯度下降优化器进行模型训练。
最后,进行预测并绘制出真实数据和预测结果的对比图。
'''
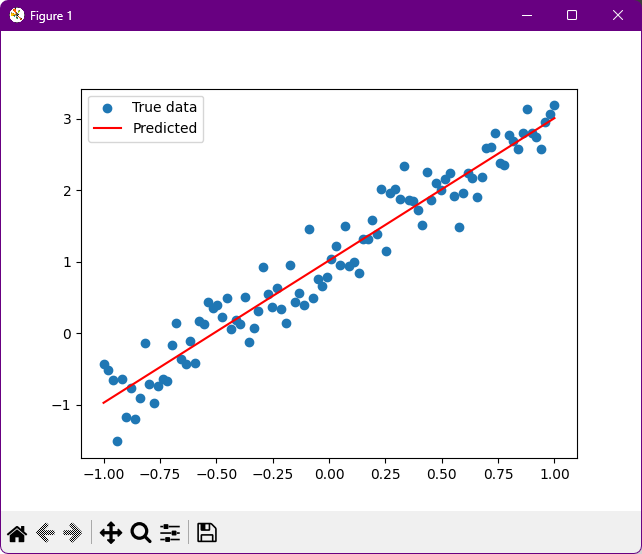
#使用 PyTorch 在 GPU 上进行线性回归的代码实例
import torch
import torch.nn as nn
import torch.optim as optim
# 设置设备为 GPU(如果可用)
device = torch.device("cuda" if torch.cuda.is_available() else "cpu")
print(f'Using device: {device}')
# 生成模拟数据
torch.manual_seed(42)
x = torch.randn(100, 1).to(device)
y = 3 * x + 2 + torch.randn(100, 1).to(device)
# 定义线性回归模型
class LinearRegression(nn.Module):
def __init__(self):
super(LinearRegression, self).__init__()
self.linear = nn.Linear(1, 1)
def forward(self, x):
return self.linear(x)
model = LinearRegression().to(device)
# 定义损失函数和优化器
criterion = nn.MSELoss()
optimizer = optim.SGD(model.parameters(), lr=0.01)
# 训练模型
num_epochs = 1000
for epoch in range(num_epochs):
# 将数据和模型都移到 GPU
inputs = x.to(device)
targets = y.to(device)
outputs = model(inputs)
loss = criterion(outputs, targets)
optimizer.zero_grad()
loss.backward()
optimizer.step()
if (epoch + 1) % 100 == 0:
print(f'Epoch [{epoch+1}/{num_epochs}], Loss: {loss.item()}')
# 在 GPU 上进行预测
with torch.no_grad():
test_input = torch.tensor([[4.0]]).to(device)
predicted = model(test_input)
print(f'Predicted value for input 4.0: {predicted.item()}')
'''
在这个例子中,首先检查是否有可用的 GPU,如果有则将设备设置为 GPU。
然后生成模拟数据并将其移到 GPU 上。定义线性回归模型并将其也移到 GPU。
使用均方误差损失函数和随机梯度下降优化器进行模型训练。最后,在 GPU 上进行预测并输出结果。
'''
[Running] python -u “d:\python\torch_gpu.py”
Using device: cpu
Epoch [100/1000], Loss: 0.917326033115387
Epoch [200/1000], Loss: 0.7827349305152893
Epoch [300/1000], Loss: 0.7804043292999268
Epoch [400/1000], Loss: 0.7803614735603333
Epoch [500/1000], Loss: 0.7803605794906616
Epoch [600/1000], Loss: 0.7803605794906616
Epoch [700/1000], Loss: 0.7803606390953064
Epoch [800/1000], Loss: 0.7803606390953064
Epoch [900/1000], Loss: 0.7803606390953064
Epoch [1000/1000], Loss: 0.7803606390953064
Predicted value for input 4.0: 14.082897186279297
[Done] exited with code=0 in 2.915 seconds